Research
Algorithms
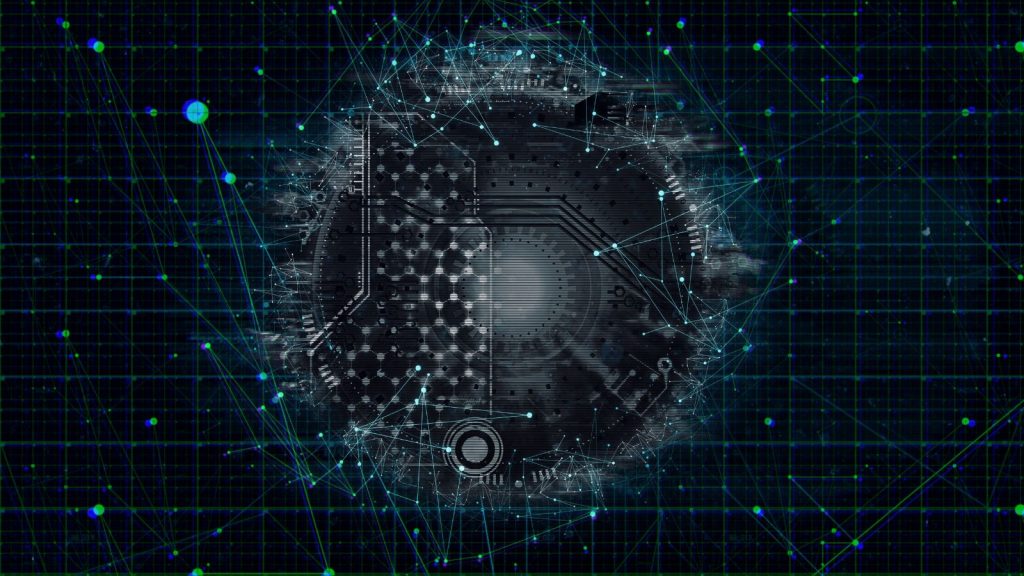
研究テーマ
効果的・効率的なアルゴリズムの実現
チームのミッション
Algorithmsチームは広告配信サービス・推薦システム・検索エンジンの最適化に関する研究開発を行っています。ユーザの行動ログに基づいて効果の高いコンテンツを配信するために、大量のデータを処理・分析することは必要不可欠です。ノイズを含むユーザ行動ログから本質的な情報を取り出すデータ分析技術、大量のデータ・配信コンテンツを分析対象とするスケーラブルな最適化技術、効果を正しく測る評価方法など、あらゆる角度からの技術開発と理論研究に取り組んでいます。
さらに、広告配信やコンテンツ推薦・検索に関する学術研究だけでなくサービスへの実装を積極的に行います。Algorithmsチームは、実応用における課題発見と先端技術開発のサイクルをチーム内で実践し、本質的な課題を解決することに挑戦します。
プロジェクト
ランキング学習
ランキング学習は、ユーザの過去の行動ログからユーザの選好を学習することで最適なコンテンツのランキングを提供することを可能にします。大量のコンテンツからなるランキングを学習するためには、学習・最適化時の計算効率を考慮する必要があります。実用的なランキングシステムを構築するにあたって、目的関数を効率的に近似する損失設計やサンプリング技術、現代的なコンピュータアーキテクチャに適した並列性を達成する最適化手法を開発しています。また、大規模なコンテンツを扱うためには大量の行動ログを活用する必要がある一方で、集めやすいクリックなどの行動ログデータは部分的にしか選好を反映せず、雑音を含むことさえあります。このようなデータを効率的かつ効果的に活用して頑健かつスケーラブルなランキング学習を実現する技術を研究しています。
多様性を考慮した推薦システム
多くの推薦システムは、ユーザの選好の推定値に基づきコンテンツを推薦するため、ユーザ選好の予測精度向上が重視されています。しかし、精度向上のみを優先させると、推薦されたコンテンツ同士が似かよってしまい、ユーザの満足度が低下する恐れがあります。このような問題を解決するため、私たちは推薦結果に「多様性」を導入する技術を研究しています。特に、「行列式点過程」と呼ばれる多様性を表す確率的モデルを利用した推薦手法について、推薦結果への影響・スケーラビリティの向上・計算の複雑性を実験的・理論的な観点から解析しています。
保守的なオンラインランキング学習(safe online learning to rank)
オンラインランキング学習では、過去に配信したコンテンツに対するユーザーのフィードバックを利用して、ユーザーの選好をリアルタイムで学習します。近年では、将来的にユーザーの選好をより正確に学習するために、敢えてユーザーが好むかどうか不明なコンテンツも配信するなど、「探索」の要素を学習アルゴリズムに加えることが多くなっています。しかし、これらのアルゴリズムの多くは、学習初期にユーザーの選好を無視してコンテンツを提供しがちであり、アプリケーションのユーザー体験を大きく損なう危険が伴います。
この問題を解決するため、探索要素の無い従来のオンラインランキング学習のランキング結果を活かしつつ、まだ掴めていないユーザーの選好を探索する、「保守的なオンラインランキング学習手法」の研究、応用に取り組んでいます。
研究領域
組合せ最適化 / 連続最適化 / 情報検索 / 推薦システム
所属メンバー
論文一覧
-
Asymptotically Optimal Inapproximability of Maxmin k-Cut Reconfiguration
理論計算機科学
52nd EATCS International Colloquium on Automata, Languages, and Programming (ICALP 2025)
-
Yet Another Simple Proof of the PCRP Theorem
理論計算機科学
52nd EATCS International Colloquium on Automata, Languages, and Programming (ICALP 2025)
-
On Approximate Reconfigurability of Label Cover
理論計算機科学
Information Processing Letters
-
Safe Collaborative Filtering
推薦システム
ICLR 2024
-
Matroid Semi-Bandits in Sublinear Time
Machine Learning
40th International Conference on Machine Learning (ICML 2024)
-
Regular Expressions with Backreferences and Lookaheads Capture NLOG
理論計算機科学
51st EATCS International Colloquium on Automata, Languages, and Programming (ICALP 2024)
-
Optimal PSPACE-hardness of Approximating Set Cover Reconfiguration
理論計算機科学
51st EATCS International Colloquium on Automata, Languages, and Programming (ICALP 2024)
-
Alphabet Reduction for Reconfiguration Problems
理論計算機科学
51st EATCS International Colloquium on Automata, Languages, and Programming (ICALP 2024)
-
Computational complexity of normalizing constants for the product of determinantal point processes
理論計算機科学
Theoretical Computer Science
-
Scalable and Provably Fair Exposure Control for Large-Scale Recommender Systems
機械学習
WWW 2024
-
Probabilistically Checkable Reconfiguration Proofs and Inapproximability of Reconfiguration Problems
理論計算機科学
56th Annual ACM Symposium on Theory of Computing (STOC 2024)
-
On the Parameterized Intractability of Determinant Maximization
理論計算機科学
Algorithmica
-
Gap Amplification for Reconfiguration Problems
理論計算機科学
35th Annual ACM-SIAM Symposium on Discrete Algorithms (SODA 2024)
-
Fast and Examination-agnostic Reciprocal Recommendation in Matching Markets
推薦
RecSys 2023
-
Toward Verifiable and Reproducible Human Evaluation for Text-to-Image Generation
推薦
CVPR 2023
-
Exploration of Unranked Items in Safe Online Learning to Re-Rank
推薦
SIGIR2023
-
Some Inapproximability Results of MAP Inference and Exponentiated Determinantal Point Processes
理論計算機科学
Journal of Artificial Intelligence Research
-
Curse of “Low” Dimensionality in Recommender Systems
推薦
46th International ACM SIGIR Conference on Research and Development in Information Retrieval (SIGIR 2023)
-
A Critical Reexamination of Intra-List Distance and Dispersion
推薦
46th International ACM SIGIR Conference on Research and Development in Information Retrieval (SIGIR 2023)
-
iMixer: invertible, implicit, and iterative MLP-Mixer from modern Hopfield networks
機械学習
MIRU 2023
-
iMixer: hierarchical Hopfield network implies an invertible, implicit and iterative MLP-Mixer
Machine Learning
arXiv プレプリント
-
Attention in a family of Boltzmann machines emerging from modern Hopfield networks
Machine Learning
Neural Computation
-
Gap Preserving Reductions Between Reconfiguration Problems
理論計算機科学
40th International Symposium on Theoretical Aspects of Computer Science (STACS 2023)
-
On Reconfigurability of Target Sets
理論計算機科学
Theoretical Computer Science
-
公平性を考慮した大規模推薦システム
機械学習
IBIS 2022
-
Fair Matrix Factorisation for Large-Scale Recommender Systems
推薦
RecSys 2022 FAccTRec Workshop
-
On the Parameterized Intractability of Determinant Maximization
理論計算機科学
33rd International Symposium on Algorithms and Computation (ISAAC 2022)
-
Matching Theory-based Recommender Systems in Online Dating
情報検索
RecSys 2022
-
AxIoU: An Axiomatically Justified Measure for Video Moment Retrieval
コンピュータビジョン
CVPR 2022
-
Optimal Correction Cost for Object Detection Evaluation
コンピュータビジョン
CVPR 2022
-
Alleviating Cold-Start Problems in Recommendation through Pseudo-Labelling over Knowledge Graph
推薦
WSDM 2021
-
Scalable Personalised Item Ranking through Parametric Density Estimation
推薦
SIGIR 2021
-
Density-Ratio Based Personalised Ranking from Implicit Feedback
推薦
WWW 2021